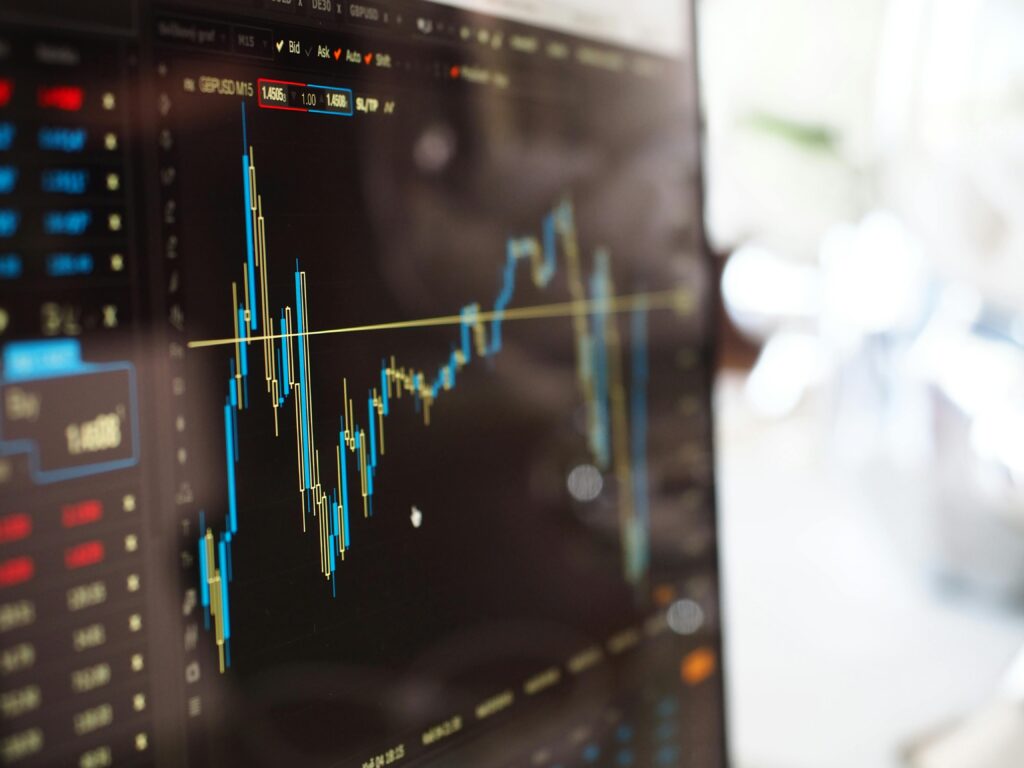
Generative interfaces can provide insights at remarkable speeds. A strategy consultant from Toptal, with a background at Deloitte and AWS, discusses how businesses of all sizes can harness Generative AI for sales, development, and customer support.
As organizations expand and adapt, they accumulate vast amounts of data, which can become fragmented and challenging to utilize effectively. Recent studies indicate that knowledge workers spend over eight hours each week merely locating and interpreting the information necessary for their tasks. However, generative artificial intelligence (Gen AI) offers a solution. With a decade of experience in consulting on digital transformation and AI integration in large enterprises, I have witnessed the significant impact Gen AI can have on optimizing knowledge management and enhancing productivity.
One of the most promising applications of AI for improving operational efficiency is through generative business interfaces. These tools enable users to search company databases using a conversational approach that does not rely on exact keywords (often referred to as semantic or conversational search). Powered by large language models (LLMs) and utilizing natural language processing (NLP), these interfaces better understand user intent compared to traditional search methods. They can generate personalized responses by synthesizing relevant information from various data sources, and some can even create new content based on the company’s data. According to McKinsey & Company, Gen AI could boost efficiency in the banking sector by up to 5%, translating to cost savings of $200 billion to $300 billion across the industry.
Numerous major enterprise resource planning (ERP) providers, such as Microsoft Copilot, Salesforce Einstein, and HubSpotAI, offer generative interfaces that integrate with their platforms and others. Additionally, various third-party solutions are available, including Coveo, Bloomreach, and Algolia. New entrants like Glean, which secured over $200 million in funding in March 2024 at a $2.2 billion valuation, and Hebbia, which raised $130 million in July 2024 at a $700 million valuation, are also making waves in this space.
A Google study indicates that leaders believe generative AI can enhance operational efficiency across creative, customer service, commerce, and supply chain functions.
SaaS vs. API: Choosing the Right Generative Interface
Third-party solutions are available as either Software as a Service (SaaS) offerings with dedicated search portals or as application programming interfaces (APIs) that can be integrated into existing systems by a company’s engineers. SaaS platforms are ideal for organizations looking to implement generative interfaces quickly with basic, out-of-the-box features for routine tasks.
Conversely, APIs are better suited for companies requiring highly customized solutions or those wanting their tools trained on specialized LLMs for greater accuracy. API-based offerings are commonly used for product search and discovery, allowing businesses to incorporate generative interfaces into their existing applications while offering advanced model training and customization options. However, this approach necessitates skilled developers and data scientists for implementation.
Pricing varies widely, with options available at all levels. Some vendors offer a freemium model that allows free usage up to a certain limit of search queries. Beyond the free tier, most vendors provide subscription or pay-as-you-go pricing, ranging from a few hundred dollars per month for small businesses to fully customized enterprise solutions that can cost hundreds of thousands of dollars annually.
Depending on the provider, companies may choose from various LLM databases, such as Anthropic’s Claude, OpenAI’s GPT-4, Facebook’s LLaMA, or Google’s Gemini. Each offers distinct advantages; for instance, GPT-4 is known for its power and versatility, while Gemini excels in integration within the Google ecosystem. The choice should be guided by the specific use case.
A key technology utilized by these models is retrieval-augmented generation (RAG), which provides real-time insights based on the latest available information and ensures traceability to original sources for easy verification.
Some providers may also use bespoke LLMs trained on highly specific datasets, customized vector databases that leverage machine learning for information categorization, and the deep learning capabilities of neural search to enhance the accuracy of search results and summaries for targeted audiences.
How AI Enhances Operational Efficiency
In general, AI can automate routine or administrative tasks, thereby boosting productivity. AI-enabled search interfaces empower employees to locate relevant information that may be siloed across disparate databases or challenging to interpret. Generative interfaces can even tailor responses based on the user’s identity and data access level. While this may not directly lower operational costs, it allows employees to concentrate on strategic activities that drive results.
Generative interfaces can benefit any department managing substantial amounts of information, such as human resources, inventory management, and supply chain management. However, in my experience, developers, sales professionals, and customer support agents are likely to see the most immediate advantages. Here’s how:
Breaking Down Knowledge Silos for Developers
Software teams are essential to the growth of many enterprises. Maximizing